5 steps to an improved data quality assurance plan
Follow these steps to develop a data quality assurance plan and management strategy that can help identify data errors before they cause big business problems.
Forward-thinking business executives recognize the value of establishing and institutionalizing best practices...
Enjoy this article as well as all of our content, including E-Guides, news, tips and more.
for enhancing data usability and information quality as part of the overall data management process. But problems can arise if companies make piecemeal investments in data cleansing and error correction. The absence of comprehensive data quality assurance and management processes leads to replicated efforts and increased costs; worse, it impedes the delivery of accurate and consistent information to business users and analytics teams.
What's needed is a practical approach for aligning disparate data quality activities with one another to create an organized program that addresses the challenges of ensuring high quality levels. Engaging business sponsors and developing a business case to justify investments are both initial requirements in creating a data quality assurance plan. But aside from them, the following five tasks and procedures are fundamental to effective data quality management and improvement efforts in organizations.
Document data quality requirements and define rules for measuring quality. In most cases, data quality levels are related to the fitness of information for business uses. Begin by collecting requirements: Engage business users, gain an understanding of their business objectives and solicit their expectations for data usability. That information, combined with shared experiences about the business impact of data quality issues, can be translated into rules for measuring key dimensions of quality, such as data completeness, currency and freshness; the consistency of data value formats in different systems and with defined sources of record should also be measured. As part of this process, document the requirements and associated rules in a centralized system to support the development of data validation mechanisms.
Assess new data to create a quality baseline. A repeatable process for statistical data quality assessment helps to augment the set of quality-measurement rules by checking source systems for potential anomalies in newly created data. Statistical analysis and data profiling tools can scan the values, columns and relationships in and across data sets, using frequency and association analyses to evaluate data values, formats and completeness and to identify outlier values that might indicate errors.
In addition, profiling tools can feed information back to data quality and data governance managers about things such as data types, the structure of relational databases and the relationships between primary and foreign keys in databases. The findings can then be shared with business users to help develop the rules for validating data quality downstream in different database management systems and other data stores.
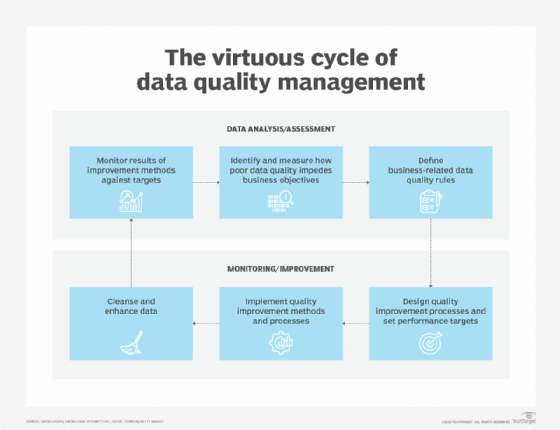
Implement semantic metadata management processes. As the number and variety of data sources grows and data integration processes connect more of them, there's a corresponding need to limit the risk that end users in different parts of an organization will misinterpret the meanings of common business terms and data concepts. To reduce the situations in which inconsistent interpretations lead to data quality and usage problems, centralize the management of business-relevant metadata and enlist business users and data management practitioners to collaborate on establishing corporate data standards. The metadata and an associated data dictionary can then be made accessible as part of a data catalog that helps users find and understand available data.
Check data validity on an ongoing basis. Develop automated services to validate data records against the quality rules you've defined. A strategic implementation enables the rules and validation mechanisms to be shared across applications and deployed at various locations in an organization's information flow for continuous data inspection and quality measurement. The results can be fed into a variety of reporting schemes -- for example, notifications and alerts sent directly to data stewards to address acute anomalies and high-priority data flaws, and dashboards and scorecards with aggregated data quality metrics for a wider audience.
Keep on top of data quality problems. Develop a platform to log, track and manage data quality incidents. Measuring compliance with your data quality rules won't lead to improvements unless there are standard processes for evaluating and eliminating the root causes of data errors. An incident management system can automate key processes, such as reporting and prioritizing data quality issues, alerting interested parties, assigning data quality improvement tasks and tracking the progress of remediation efforts.
Done properly, these activities form the backbone of a proactive data quality assurance plan and management framework, with controls, rules and processes that enable an organization to identify and address data flaws before they cause negative business consequences. In the end, fixing data errors and inconsistencies and making sure their root causes are dealt with will enable broader and more effective utilization of data, to the benefit of your business.